Are you using Facebook and WhatsApp? Are you still using them?
Early this year, people started to migrate from Facebook and WhatsApp to MeWe, Signal and Telegram. Approximately 7.5 millions new downloads for Signal are recorded in just 5 days. What is causing this phenomenon and what does it mean for businesses?
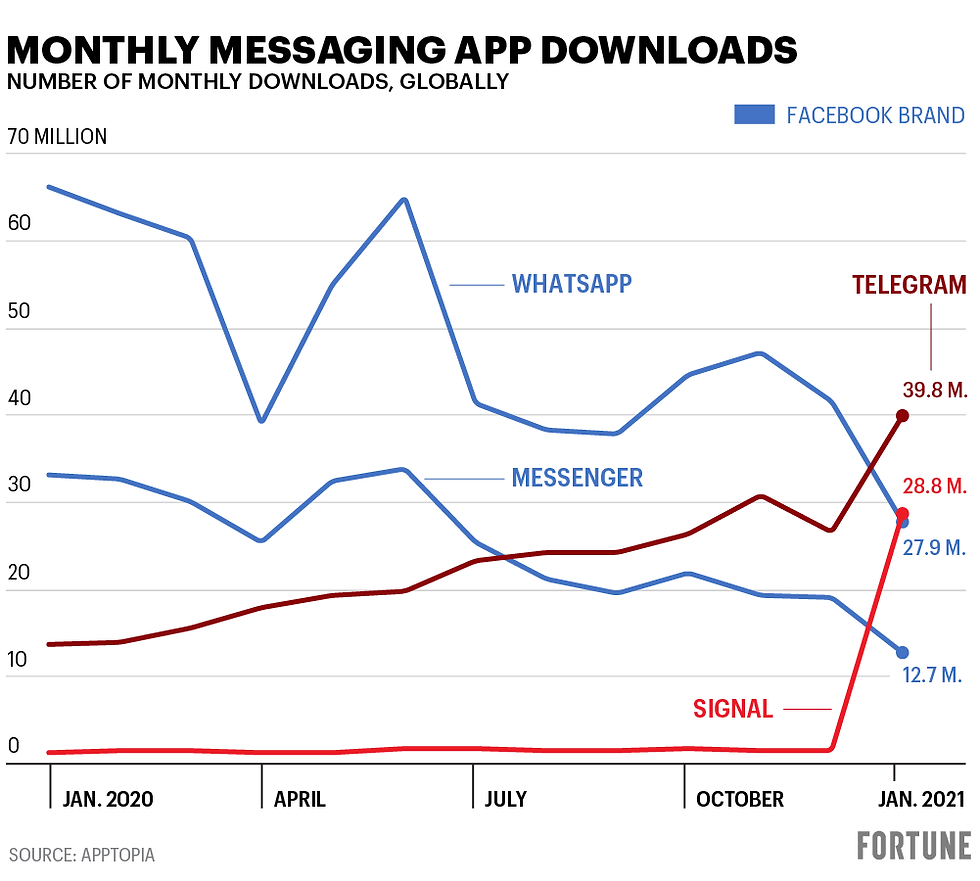
Increasing concerns about data privacy on social platforms
People migrate from one social platform to another due to different reasons. Some switch because their friends are switching. It would be hard not being able to communicate to your friends if most of them are using a platform that you are not using. The "Network Effect" can explains this, where the more users a social platform has, the more content it holds; the more content it holds, the more value the platform offers.
Some others are changing for privacy concerns. Right after WhatsApp announced that it would share user data with Facebook, people fled from the app and sought alternatives, thus the booming increase in downloads of Signal and Telegram, which claim to provide better user data security.
The rise in awareness of data privacy has made social platform users more cautious of their personal information. With the increase of "personalised" ads and content on social media (e.g. ads on Instagram about the phone case a user just browsed on Amazon), users are getting more aware of how social platforms are using their personal information.
Connect the dots across social platforms with user data
User data is the reason that most social platforms like Facebook are free. In the past, users paid for the platform they use. Now with most platforms being free, users don't actually realise they are giving out personal data in exchange for the platforms' free services. (Yet, there are paid platforms that also sell users' data to third parties.)
“If you’re not paying for the product, then you are the product.” — Daniel Hövermann
User data is crucial to social platforms. It has enabled platforms to provide better user experiences, providing more relevant and personal content. By utilising data such as demographics and preferences, social platforms can push content to the right audiences who have a bigger interest in it than others. The more these social platforms understand their users, the more the content can be tailored to each user's taste and interest.
This is why Facebook purchased WhatsApp in 2014, even if WhatsApp was losing a lot of money then. A user may never talk or interact with a friend on Facebook, but they might talk a lot on WhatsApp. Combining user behaviours on both Facebook and WhatsApp will provide a full picture of how users are related to each other.
Understand user relationships with Social Network Analysis
When relationships between users are drawn on paper, it becomes a social graph. A social graph represents how a user and friends are connected. It helps social platforms to know which social circles a user is in, who are their best friends, and how they interact.
For instance, if the platform identifies a user's main social circle is formed by fashion-forward shoppers, then the user is likely to be a fashionista as well. A user's social circle will define who the user is and how they behave.
Take the social graph below as an example: A social platform knows that user X is a friend of user A and another user in the art lover community in blue. The social platform will likely recognise user X as an art lover and expect her to have similar behaviours as the other art-loving users.
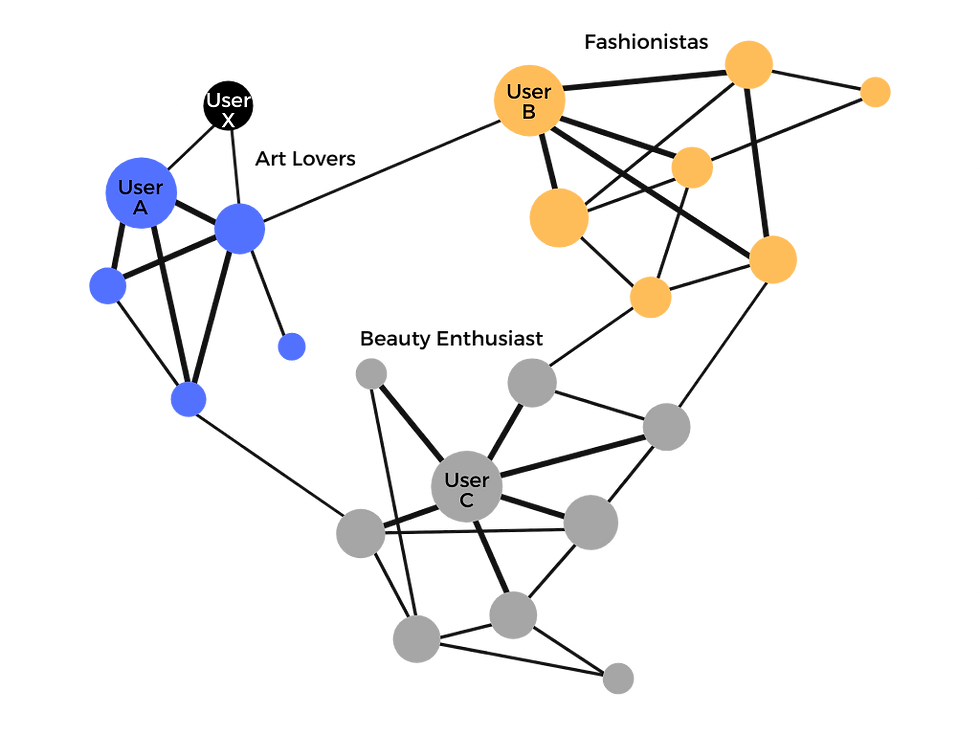
This is the foundation of social network analysis (SNA). It allows social platforms to locate target audiences, even if a user has never posted anything online. From a user's social graph, platforms can infer the user's age, the movies the user might like, the places the user might usually go and much more other information.
How to leverage SNA to optimise your business
Social network analysis can do much more than just knowing the background and preferences of a user. With SNA, you can detect communities, locate people who have the most social influences and even predict the next best-selling products.
To predict whether your product will go viral, you only need to take a look at the first 100 people who shared your post on social media. If the users who shared are coming from many different communities on a social graph, it is more likely that the product will go viral.
SNA is also capable to detect an important user, say a KOL, who can lead the trends. If a retailer is able to pinpoint the most relevant KOL for campaigns, the company can be smarter on its marketing budget.
SNA can also predict the timing of virality and the number of "shares" a post can reach. This empowers retailers to dynamically adjust the resources based on the expected campaign performance and the targeted number of "shares" for the post.
Customer centricity is the key when utilising customer data
Social network analysis can bring business value to retailers by detecting important opinion leaders within a group of customers and even helping to curate ad contents that are relevant to a brand's audiences. Leveraging social network analysis can improve the effectiveness of marketing strategies and overall product design.
However, every coin has two sides. While businesses collect, analyse, and use data, it is important to remain customer-centric: you should not be collecting data simply for the sake of data enrichment, but to make good use of it to ultimately improve customer experience.
After all, you want to acquire and retain customers rather than pushing your customers to your competitors right?
References:
[1] Kharpal, Arjun. "Signal and Telegram downloads surge after WhatsApp says it will share data with Facebook."
[2] Weng, Lilian, Filippo Menczer, and Yong-Yeol Ahn. "Virality prediction and community structure in social networks." Scientific reports 3.1 (2013): 1-6.
[3] Pei, Sen, et al. "Searching for superspreaders of information in real-world social media." Scientific reports 4.1 (2014): 1-12.
[4] Cheung, Ming, et al. "Prediction of virality timing using cascades in social media." ACM Transactions on Multimedia Computing, Communications, and Applications (TOMM) 13.1 (2016): 1-23.
Comentários